Detection and Separation of Recyclable Plastics from Municipal Solid Waste (Year 2)
[This website is for the 2nd year of the project entitled “Detection and Separation of Recyclable Plastics from Municipal Solid Waste.” (Year 1)]
The project aims to prototype a portable system for polymer resin identification based on mid-infrared (MIR) spectroscopy. This system, along with the multispectral fingerprint detection mechanism currently under development, would serve as a prototype for further integration into more specialized solutions for recycling and recovery applications. For example, the system could be located at material recovery facilities (MRF), end-user receiving sites, recycling kiosks, etc.
In addition, two aspects of recovered plastics will be studied during this 2nd year work: plastic cross-contamination between different resins and plastic contamination from typical contaminants found at MRF. With the high-end commercial MIR spectrometer system currently available, this hand-held detection system will provide further solutions to current ever-growing problems faced in the recycling and waste management businesses.
Motivation
The US EPA estimated that among 258 million tons of municipal solid waste (MSW) generated in 2014; plastic accounted for about 33 million tons (1). Worldwide plastics represent 35% of MSW. Despite a focused effort on diverting plastics from the waste stream, recycling rates in the US for PET bottles are 31.3% and for HDPE, 28.2% (1). The additional 14 plastic types commonly found in MSW are recovered and recycled at much lower rates. Recovery and recycling of plastics is important because plastic waste is generated at high rates (mostly from single-use objects), it relies largely on a nonrenewable material for production, it has high energy content, and, if placed in a landfill or improperly disposed of, plastic will resist degradation for centuries (2). Communities are providing more opportunities to separate plastics and appropriately dispose of them to increase recovery and recycling efficiency. These typically rely on the consumer to make correct decisions regarding disposal. The ability to more efficiently identify and communicate the resin used to produce the plastic object will ensure maximum accuracy in disposing of waste materials whether it is at the curb, at a receptacle in shopping centers, institutions, or business, or while separating materials in a materials recovery facility.
Goals and Objectives
The goal of this project is to translate the laboratory-proven method for detecting and differentiating polymer resins to a field deployable system and to test this system under field conditions. The key objectives of the research are:
- Adapt the laboratory setup to a robust field-compatible configuration,
- Gather plastic spectral data using the new configuration
- Evaluate classification algorithms including neural networks for machine-automated identification of plastic category.
This project will provide a fieldable new technology for the detection and separation of multiple types of plastics with direct application to the recycling process. The technology will improve the effectiveness of recycling and increase diversion from landfills especially for plastics without a RIC. With improved detectability, the plastic types that are poorly recycled can be recovered at greater rates (e.g., plastic film, polystyrene, polypropylene), ensuring a more reliable stream for plastic markets.
Scope of Work
Task 1. Infrared spectrometer integration in field adapted device. The laboratory-scale measurement setup based on MIR-FTIR spectroscopy has proven to provide reliable identification of unknown plastic resins. The aim of this task is to adapt the previously demonstrated concept in a compact footprint and, at the same time, ensure the high signal to noise ratio needed for accurate identification of contaminated plastic samples is available under field conditions. This task requires design of a higher numerical aperture optical system for delivery and collection of the optical signal and high-power IR source. While the FTIR spectroscope produces high-resolution reflectance spectra, it does so at a high cost, both monetarily and in system complexity. It also uses a low power illumination source since it is built for a closed laboratory environment. Adapting it to field conditions has proven a bit difficult task primarily due to the low power IR sources and absorption of light in the long remote fiber.
However, the 1st year work helped us to envision and design a viable alternative. The proposed optical system schematic is shown in the Figure 1. The sample to be identified is illuminated by a high-intensity broad-band IR source. The reflected light is captured by a spectrometer, which in this case is a dispersive prism and slit arrangement. The collecting optics of the spectrometer gathers the reflected light and collimates it into a small beam. This beam is spectrally spread by the prism and reflected off a mirror into the detector. As the mirror rotates, different wavelength ranges pass through the slit to the detector. As the mirror scans, detector output provides a spectral signature of the reflected light. Finally, the signal is processed in the control unit to extract the reflection spectrum and perform the automated classification. The classification is based on the retrieved chemical information from the MIR spectrum as demonstrated in our preliminary lab scale demonstration and to be augmented in Task 2.
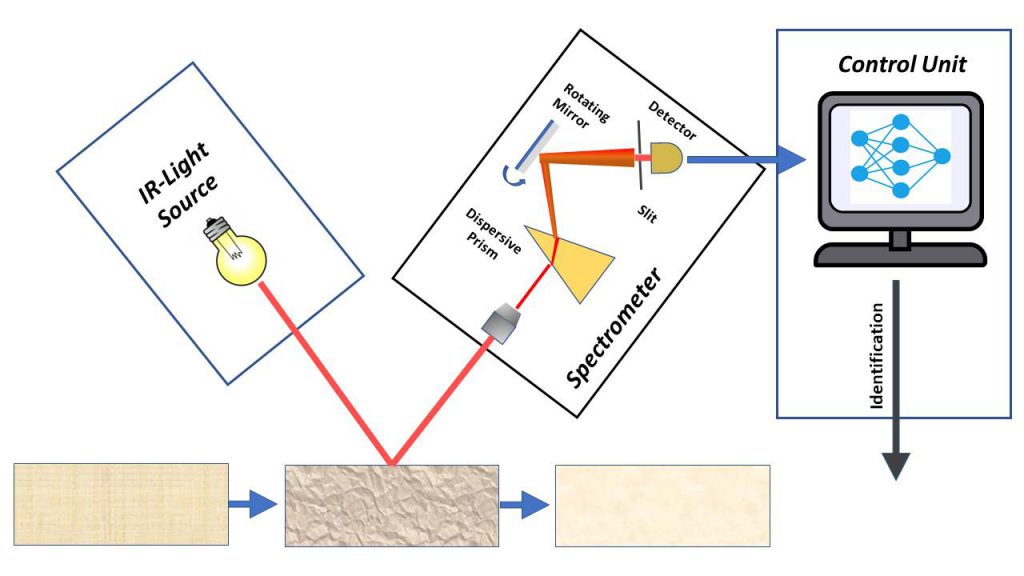
Task 2. Sample collection. The objective of this task is to evaluate the efficiency of our proposed field-able hand-held device under different types of plastic resins. Plastic samples have already been collected from UCF offices and research team homes and continue to be collected. At least fifty samples of each polymer will be desired in order to provide a representative sample for the plastic spectral library. Items will remain as is without further sample preparation in order to account for common contaminants mixed, for example, during the disposing process. Two types of contaminations will be determined: mixed plastic contamination and external material residue contamination. The ability of the MIR-FTIR system to differentiate plastic types will be compared before and after cleaning. This will enable us to establish spectral fingerprints of various plastic types in presence of contaminants. In addition, our existing plastic library will be expanded to account for the diversity of the samples, especially fillers, and to establish the detection tolerances.
Task 3. Optimization of the identification algorithm. The concepts of using a low spectral resolution spectrometer and training a neural network to discriminate plastic categories is supported by the current effort. The wavelength averaging process used to generate the features illustrated in Figures 5 and 6 can be interpreted as passing a simulated slit over this data at 100 locations. These early simulation results lend credibility to low resolution spectral sampling with a slit and neural network automation of classification. The neural network in this new task will be trained on data acquired using the new prism/slit setup. This neural network will then be optimized by modifying the structure and training methods and testing on data not used in the training process. Such machine learning techniques help produce a system that is highly accurate and robust.
Task 4. Iterative system improvement. This task goes beyond the optimization in task 3 by addressing possible improvements to the system as an integrated whole. The layout of the spectrometer shown in Figure 8 does not indicate the intricate set of design tradeoffs made among numerous system parameters. For example, using a larger slit width improves signal-to-noise ratio, but decreases wavelength discrimination. A beam-limiting aperture between the collimating optics and the prism (not shown in Figure 8) lowers signal-to-noise but decreases spectral blurring. The nonlinear relationship between refraction index and wavelength causes dispersion at 12 microns to be decreased by a factor of 1.8 compared to the dispersion at 2 microns, so the discrimination of spectral signature features will be reduced by this factor. On the other hand, the spectral signature at 1 micron is dispersed by a factor of 8 over the dispersion at 2 microns, so discrimination of NIR spectral characteristics is correspondingly greatly enhanced. These are only a few of the parameters that may be modified and each one will affect the training of the automated classifier. In response to each of the modifications of the spectrometer, the training parameters of the neural network classifier may need to be modified as well. The anticipated result is the specification of a robust system that can perform well in the field.
Task 5. Market Analysis. This task will require market exploration and development of a business plan. We will work with the UCF Venture Accelerator which offers an array of business advisory services that are geared towards helping entrepreneurs gain the business skills needed to commercialize research.
Task | Q1 | Q2 | Q3 | Q4 |
Task 1: Infrared spectrometer field device prototype. | ✔ | ✔ | ||
Task 2: Sample collection. | ✔ | ✔ | ✔ | ✔ |
Task 3: Identification algorithm upgrade | ✔ | ✔ | ✔ | |
Task 4: Iterative system improvement. | ✔ | ✔ | ||
Task 5: Market Analysis | ✔ | ✔ |
Progress Reports
TAG Meetings
Second TAG meeting
May 6, 2020 over ZOOM
Second Tag Meeting Video Recording along with the corresponding PPT presentation.